Sign up to our newsletter Subscribe
Establishing the Economic Value of Carbon-Minimal Inhalers
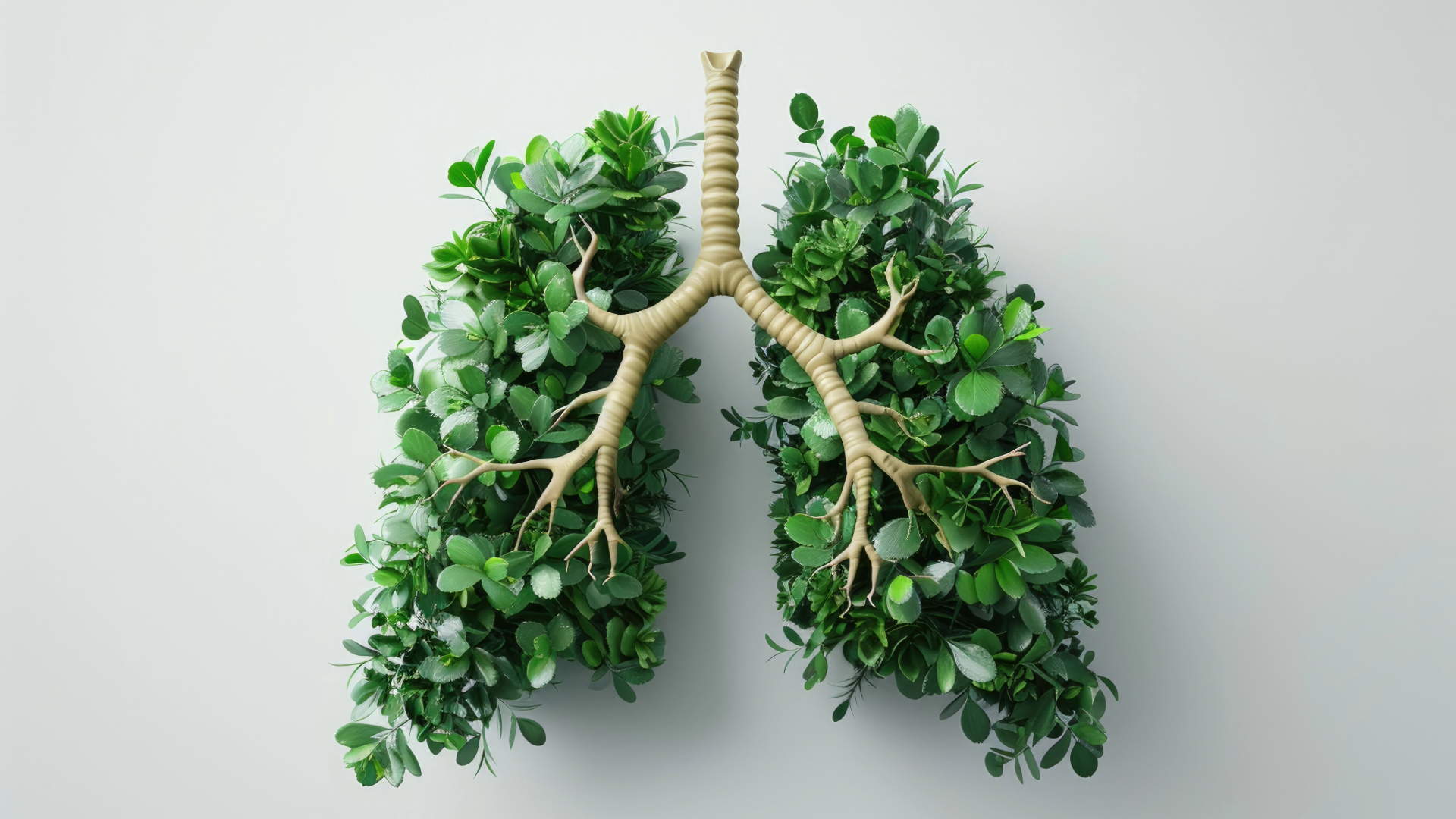
Sign up to our newsletter Subscribe
An exploration of the non‐iterative time trade‐off method to value health states – our new paper published in Health Economics Preference‐based health state classification systems require health state valuations. The conventional time trade‐off (TTO) method usually relies on ‘iteration’, which…
An exploration of the non‐iterative time trade‐off method to value health states – our new paper published in Health Economics
Preference‐based health state classification systems require health state valuations. The conventional time trade‐off (TTO) method usually relies on ‘iteration’, which is susceptible to bias. Discrete choice experiment with duration (or DCETTO) is free of such bias, but respondents find this cognitively more challenging. Furthermore, the conventional TTO method separates protocols depending on whether the health state is better or worse than being dead, and data for the latter are typically subjected to arbitrary transformation. The lead time TTO approach has been suggested, which allows the shorter duration in full health to take negative values and requires no arbitrary transformation of data. However, respondents may ‘exhaust’ lead time.
In our newly published paper in Health Economics, we explore the feasibility of the non-iterative TTO (NITTO) method with or without lead time. The NITTO without lead time is a cross between iterative TTO and DCETTO. The motivations are to avoid the iteration bias of TTO and to reduce some of the cognitive burden associated with DCETTO. A possible adaptation of the NITTO method is to add lead time to the NITTO scenarios, resulting in the non-iterative lead time TTO method (NILTTTO).
We conducted four experimental tasks including DCETTO, NITTO, NI(LT)TTO (with a single step design to select pairs of health scenarios) and NI(LT)TTO-II (with a two-stage design to select pairs of health scenarios). Each is presented in two modes of presentations, i.e. a tabulated presentation and a visual aid presentation. In total, our study has eight survey variants.
Each of the survey variants includes 12 experimental choice tasks and a 13th task with a logically determined answer. Data on the 12 experimental choices from an online survey of 6,618 respondents are modelled, by variant, using conditional logistic regressions.
Our results indicate that the NI(LT)TTO method is feasible, but some relatively mild health states appear to have implausibly low predicted values, and the range of predicted values is much narrower than in DCETTO. The presentation of NI(LT)TTO tasks needs further improvement.
This paper is authored by Dr Yan Feng, Professor Arne Risa Hole, Dr Milad Karimi, Professor Aki Tsuchiya (Principal Investigator) and Professor Ben van Hout. Earlier versions of this paper have been presented at the EuroQol Group conference (Berlin, 2016) and the Health Economists’ Study Group meeting (Birmingham, 2017). The final version of the paper has been presented at the Aberdeen Health Economics Research Unit seminar (Aberdeen, 2018). The project is funded by the EuroQol Research Foundation.
The paper is open access and available for free download. Click here to access the paper.
Feng, Y., Hole, AR., Karimi, M., Tsuchiya, A., & van Hout, B. (2018). An exploration of the non-iterative time trade-off method to value health states. Health Economics, Early View. DOI | PubMed
An error has occurred, please try again later.
This website uses cookies so that we can provide you with the best user experience possible. Cookie information is stored in your browser and performs functions such as recognising you when you return to our website and helping our team to understand which sections of the website you find most interesting and useful.
Strictly Necessary Cookie should be enabled at all times so that we can save your preferences for cookie settings.
If you disable this cookie, we will not be able to save your preferences. This means that every time you visit this website you will need to enable or disable cookies again.
This website uses Google Analytics to collect anonymous information such as the number of visitors to the site, and the most popular pages.
Keeping this cookie enabled helps us to improve our website.
Please enable Strictly Necessary Cookies first so that we can save your preferences!