Sign up to our newsletter Subscribe
Analysing Global Immunisation Expenditure
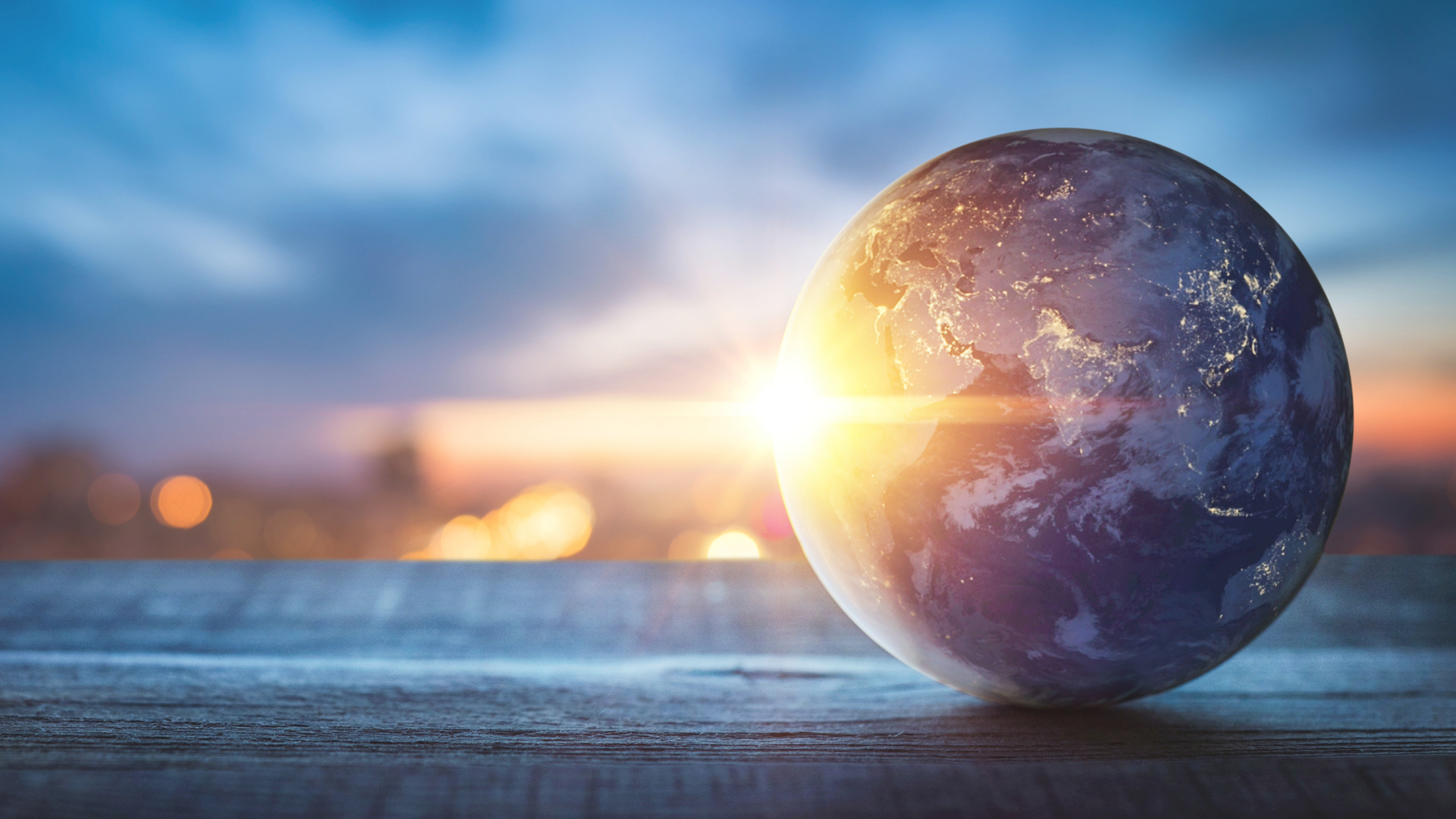
Sign up to our newsletter Subscribe
OHE and the Pharmaceutical Oncology Initiative (POI) held a workshop to discuss and debate several key issues in oncology modelling. Participating were more than 50 experts from industry, academia and government. The focus of the workshop was three key technical…
OHE and the Pharmaceutical Oncology Initiative (POI) held a workshop to discuss and debate several key issues in oncology modelling. Participating were more than 50 experts from industry, academia and government. The focus of the workshop was three key technical challenges associated with using clinical trial data for anti-cancer medicines and adapting it, in an unbiased fashion, to support decisions about cost-effectiveness.
OHE and the Pharmaceutical Oncology Initiative (POI)[1] held a workshop to discuss and debate several key issues in oncology modelling. Participating were more than 50 experts from industry, academia and government.
The focus of the workshop was three key technical challenges associated with using clinical trial data for anti-cancer medicines and adapting it, in an unbiased fashion, to support decisions about cost-effectiveness. These are: extrapolating from progression-free survival, correcting for patient crossover, and efficient modelling of different sequences of treatment.
Based on research commissioned by the POI, Prof Neil Hawkins of Oxford Outcomes discussed the problems raised by extrapolating from clinical trial data that captures “progression-free survival” to calculate “overall survival”. The latter is required to assess changes in quality adjusted life years (QALYs).
Among the issues considered were the multiple types of uncertainty that characterise clinical trial data and the dangers of naïve extrapolation or “testimation”, where observed trial data are used to select among competing model choices post hoc. Prof Hawkins called for a careful weighing of the available models for extrapolation and for involvement of health technology assessment (HTA) policy makers early in the extrapolation process.
A potentially promising approach identified by Prof Hawkins is increased collection and use of additional output data during clinical trials for the purpose of helping to solidify understanding of the complex relationships among outcomes in different trial populations. This approach ultimately may be used to develop an improved approach to modelling that integrates under-observed trial outcomes into overall survival estimates, as a way to provide regulatory authorities with the measures they need to decide whether to approve a new drug.
Prof Andrew Briggs of the University of Glasgow presented a case study that provides another perspective on overall and progression-free survival. He also suggested the use of more general methods of analysis, such as multi-state survival analysis, that permit the direct estimation of both overall survival and progression-free survival from the trial data.
Nicholas Latimer, from ScHARR at the University of Sheffield, discussed the results of a study commissioned by POI that focuses on methods for adjusting survival estimates in the presence of treatment crossover. This statistical problem arises when ethical considerations require that clinical trial participants be permitted to “cross over” to alternative branches of a trial once preliminary data has demonstrated that they would benefit from the alternative treatment. This non-random migration from the original allocation complicates analysis of the results produced in trials if a substantial degree of crossover occurs.
The ScHARR POI study is based on a simulation that used hypothetical patient groups. It was meant to test a number of statistical techniques including popular, but potentially unsound approaches, such as simply discarding data from crossed-over patients. The results of the 72 scenarios tested showed significant differences in effectiveness for the various correction methods depending on the number of patients that crossed over and whether the new treatment was less effective in patients who crossed over later in the progression of the disease. While no single “best” approach to correction was identified, certain approaches did perform poorly consistently. Determining which method is likely to produce the least bias may be possible through analysis of the treatment crossover mechanism and the clinical trial dataset.
Dr Martin Hoyle of PenTAG provided an alternative view on the problem of treatment crossover based on his direct experience in evidence review. He emphasised the importance of being pragmatic in choosing among various methods and the importance of model choice in each case.
A moderated panel discussion featured brief presentations from representatives of industry, government and academia on the difficulties of modelling treatment sequences, an issue that arises when patients in a clinical trial receive a number of treatments in different sequences. This produces data that are difficult to incorporate parsimoniously into HTA models. A general discussion that involved the audience identified existing options for extracting the effect of individual medicines from sequenced data. This will provide the starting point for a working paper to be completed for POI this year.
The workshop concluded with a panel discussion exploring how insights from the two studies conducted for POI by Oxford Outcomes and ScHARR could inform current UK HTA processes. Participating were Peter Donnan of the University of Dundee, a member of the NDC Committee of the Scottish Medicines Consortium, and Helen Knight and Meindert Boysen from NICE.
An error has occurred, please try again later.
This website uses cookies so that we can provide you with the best user experience possible. Cookie information is stored in your browser and performs functions such as recognising you when you return to our website and helping our team to understand which sections of the website you find most interesting and useful.
Strictly Necessary Cookie should be enabled at all times so that we can save your preferences for cookie settings.
If you disable this cookie, we will not be able to save your preferences. This means that every time you visit this website you will need to enable or disable cookies again.
This website uses Google Analytics to collect anonymous information such as the number of visitors to the site, and the most popular pages.
Keeping this cookie enabled helps us to improve our website.
Please enable Strictly Necessary Cookies first so that we can save your preferences!